Find out how HackerEarth can boost your tech recruiting
Learn more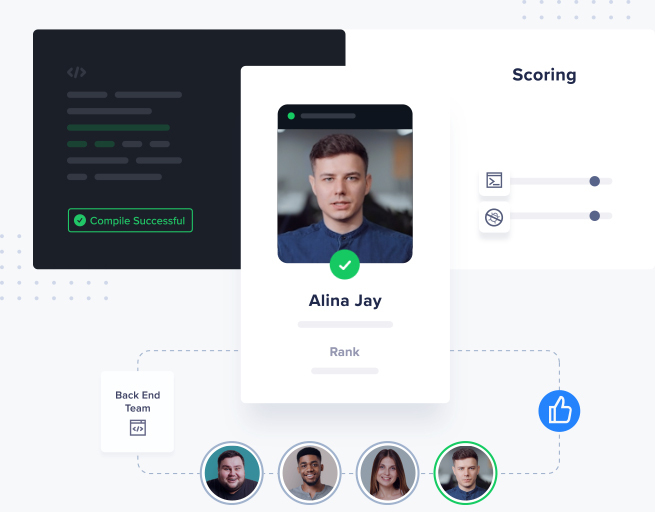
Feature Engineering + H2o Gradient Boosting (GBM) in R Scores 0.936
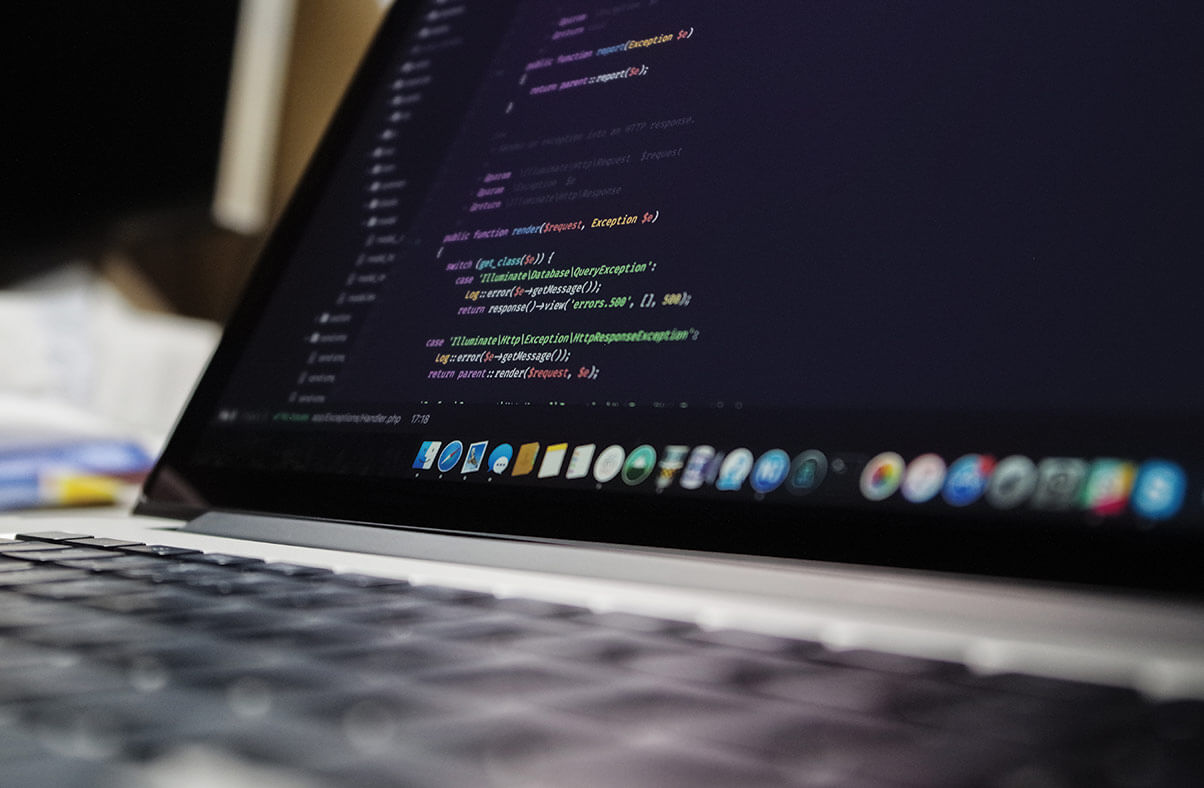
With less than 3 days to go, this script is meant to help beginners with feisty ideas, machine learning workflow and motivation for ongoing machine learning challenge.
Here’s a quick workflow of what I’ve done below:
- Load data and explore
- Data Pre-processing
- Dropped Features
- One Hot Encoding
- Feature Engineering
- Model Training
Good Luck!
Note: For more feature engineering ideas, spend time on exploring data by loan_status variable. For categorical vs categorical data, create dodged bar plots. For categorical vs continuous data, create density plots and use fill=as.factor(loan_status).
To help the community, feel free to contribute the equivalent python / C ++ script in the comments below.
Update: You can get python script for this solution from Jin Cong Ho’s comment below.
Script (R)
Resources – Handy Algorithms for this Challenge

Get advanced recruiting insights delivered every month
Related reads
The complete guide to hiring a Full-Stack Developer using HackerEarth Assessments
Fullstack development roles became prominent around the early to mid-2010s. This emergence was largely driven by several factors, including the rapid evolution of…
Best Interview Questions For Assessing Tech Culture Fit in 2024
Finding the right talent goes beyond technical skills and experience. Culture fit plays a crucial role in building successful teams and fostering long-term…
Best Hiring Platforms in 2024: Guide for All Recruiters
Looking to onboard a recruiting platform for your hiring needs/ This in-depth guide will teach you how to compare and evaluate hiring platforms…
Best Assessment Software in 2024 for Tech Recruiting
Assessment software has come a long way from its humble beginnings. In education, these tools are breaking down geographical barriers, enabling remote testing…
Top Video Interview Softwares for Tech and Non-Tech Recruiting in 2024: A Comprehensive Review
With a globalized workforce and the rise of remote work models, video interviews enable efficient and flexible candidate screening and evaluation. Video interviews…
8 Top Tech Skills to Hire For in 2024
Hiring is hard — no doubt. Identifying the top technical skills that you should hire for is even harder. But we’ve got your…
